Decoding the Future: Natural Language Processing’s Role in Advancing IoT Applications
Introduction to Natural Language Processing (NLP)
Natural Language Processing (NLP) has emerged as a revolutionary field in the intersection of computer science, artificial intelligence, and linguistics. It focuses on enabling computers to understand, interpret, and generate human language in a way that is both meaningful and contextually relevant. NLP has paved the way for significant advancements in various industries, including healthcare, finance, and customer service. In this comprehensive guide, we will delve deep into the world of NLP, exploring its history, inner workings, applications, challenges, and future prospects.
History of Natural Language Processing
The roots of NLP can be traced back to the 1950s, when researchers began exploring the possibilities of machine translation. The advent of computers and the increasing availability of textual data sparked interest in developing algorithms that could process and analyze human language. The early years of NLP were marked by rule-based approaches, where linguists manually crafted intricate sets of rules to translate and analyze text. However, these approaches proved to be limited in their ability to handle the complexity and ambiguity of natural language.
The field witnessed a significant breakthrough in the 1980s with the introduction of statistical methods in NLP. This approach involved training models on large amounts of annotated data to learn patterns and make predictions. The rise of machine learning algorithms, such as Hidden Markov Models and Neural Networks, further propelled the progress in NLP. With the advent of deep learning and the availability of massive datasets, NLP has witnessed unprecedented growth, enabling machines to comprehend and generate human language with astonishing accuracy.
How Does Natural Language Processing work?
Natural Language Processing involves a series of intricate steps to process, analyze, and understand human language. The first step is known as tokenization, where the text is broken down into individual words or tokens. These tokens serve as the building blocks for subsequent processing steps. The next step involves part-of-speech tagging, where each word is assigned a grammatical category, such as noun, verb, or adjective. This information helps in understanding the syntactic structure of the text.
Once the text is tokenized and tagged, the next step is to analyze the relationships between words. This is done through syntactic parsing, which involves creating a parse tree that represents the grammatical structure of the sentence. Sentiment analysis is another important aspect of NLP, where the underlying sentiment or emotion expressed in the text is determined. This can be crucial for applications such as social media monitoring or customer feedback analysis.
Applications of Natural Language Processing
Natural Language Processing has found a wide range of applications across various domains. In the healthcare industry, NLP is used to extract important information from medical records, enabling faster and more accurate diagnosis. Sentiment analysis is employed in customer service to gauge customer satisfaction and identify areas for improvement. NLP algorithms are also used in automated translation services, making it easier for people to communicate across different languages and by chatbots to provide personalized assistance and support in various industries, including e-commerce and banking. In the legal domain, NLP is used for e-discovery, where large volumes of legal documents are analyzed to extract relevant information for legal professionals.
Natural Language Processing in the Internet of Things (IoT)
IoT has ushered in a new era of interconnected devices that communicate and share data with each other. NLP plays a vital role in extending this data exchange beyond device-to-device communications to deliver valuable insights to the users of these devices. Voice assistants like Amazon Alexa and Google Assistant utilize NLP algorithms to understand and respond to user commands. By leveraging NLP, IoT devices can interpret and act upon natural language instructions, making them more intuitive and user-friendly.
Voice assistants will also provide a convenient means for drivers to interact with dashcams integrated into fleet telematics solutions with neural network capabilities. Refer to our blog, “Navigating the Neural Network Wave: Unveiling New IoT Capabilities with Neural Networks and Devices” for additional details. As drivers make use of this feature, the dashcam utilizes speech recognition technology to convert spoken language into text. Subsequently, NLP algorithms are activated, carefully analyzing the transcribed text to understand and interpret the driver’s instructions or queries. This seamless fusion of voice commands and NLP elevates the driving experience, allowing for intuitive and hands-free control of dashcam functionalities.
In industrial IoT, NLP plays a crucial role in predictive maintenance. By analyzing maintenance logs, manuals, and textual data from sensors, NLP algorithms can identify patterns indicative of potential equipment failures, enabling key personnel to take more proactive maintenance measures to minimize downtime and enhance operational efficiency.
NLP also enhances the functionality of AI-enabled dashcams used in vehicle telematics solutions by accessing and analyzing dashcam footage. As an example, by analyzing the audio information in the dashcam recordings, NLP can identify and extract information related to specific incidents, such as accidents or road events. This facilitates automated incident reporting and documentation.
Challenges in Natural Language Processing
Despite the remarkable progress in NLP, several challenges persist. One major challenge is the ambiguity and complexity of natural language. Words can have multiple meanings, and context is crucial in understanding their intentions. Another challenge is the lack of labeled training data for specialized domains. NLP models often require large amounts of annotated data to achieve high accuracy, which may not be readily available for niche domains. Additionally, ethical considerations regarding privacy and bias in NLP algorithms need to be addressed to ensure fair and responsible use of the technology.
The Future of Natural Language Processing
The future of Natural Language Processing holds immense potential. As research in the field continues to advance, we can expect even greater accuracy and sophistication in language understanding and generation. NLP algorithms will become more adaptable to different domains and languages, enabling machines to understand and communicate with humans in a more natural and human-like manner. As a result, NLP will play a pivotal role in transforming industries such as healthcare, education, and customer service, making our interactions with machines more seamless and intuitive.
Conclusion
Natural Language Processing has revolutionized the way machines interact with human language, opening up a world of possibilities across various industries and domains. From its humble beginnings in machine translation to the current advancements in deep learning, NLP has come a long way. As we continue to unravel the complexities of human language, the power of NLP will continue to grow, enabling machines to understand, interpret, and generate language in ways that were once unimaginable. Embracing the potential of NLP will pave the way for a future where human-machine interactions are seamless, intuitive, and truly transformative.
About Positioning Universal
Established in 2013, Positioning Universal is a leading global provider of off-the-shelf customizable IoT devices, GPS vehicle and asset monitoring solutions, and Systems Integration services. With a deep understanding of IoT technologies, Positioning Universal guides companies in designing and deploying the most suitable IoT solutions for their needs. Our solutions and on-going support empower businesses with the invaluable business intelligence needed to maintain a competitive edge in rapidly evolving markets.
Positioning Universal Viewpoint
The next generation of telematics solutions, featuring neural network components and NLP, is set to transform user-device interaction. Drawing inspiration from the familiarity and ease of communication with voice assistants, users will be able to effortlessly manage and access telematics functionalities, from querying real-time vehicle data to issuing commands for navigation or safety features. Telematics providers will be able to provide over-the-air (OTA) updates for these devices, mirroring the approach employed by Tesla to introduce new capabilities to their vehicles. The possibilities are endless and will mark a significant advancement in making the interaction between users and their IoT devices more fluid, efficient, and responsive to individual preferences.
We continuously monitor and incorporate into our product roadmaps relevant technological advancements, including emerging developments in neural networks and NLP, in our constant quest to improve the quality of the telematics solutions we provide to our resellers and customers.
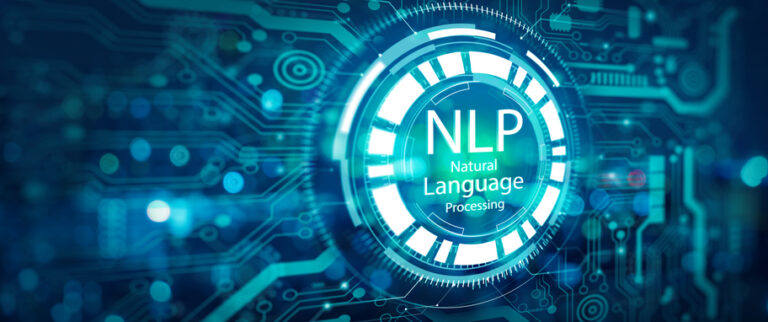
Author
Aiza LamugRelated posts
The Power of Machine Learning in Telematics for Predictive Maintenance
Introduction Telematics systems are harnessing the power of machine learning to
How Satellite Constellations Are Shaping Our World
Intro Satellite constellations comprise three distinct orbits: Geostationary Orb
Revolutionizing Connected Car Technology: How Smart PowerSM Technology is Changing the Game
The telematics industry is undergoing a transition towards the increased utiliza