The Power of Machine Learning in Telematics for Predictive Maintenance
Introduction
Telematics systems are harnessing the power of machine learning to transform vehicle maintenance from reactive to predictive. By analyzing vast amounts of real-time data, machine learning algorithms can detect patterns and anomalies that may indicate potential vehicle issues. This enables fleet managers and maintenance teams to proactively address these issues, thus avoiding costly breakdowns and minimizing downtime. With predictive maintenance, vehicle servicing can be scheduled based on actual need rather than arbitrary intervals, resulting in significant cost savings and improved operational efficiency.
Moreover, machine learning-powered telematics systems constantly learn and adapt, becoming smarter over time. They can identify various factors that contribute to vehicle wear and tear, such as driving behavior and environmental conditions, to provide accurate predictions. This data-driven approach not only enhances vehicle reliability and safety but also helps optimize fleet operations.
What is Machine Learning and How Does It Relate to Telematics?
Machine learning is a subset of artificial intelligence (AI) that enables computers to learn and make predictions or decisions without being explicitly programmed. It involves the development of algorithms that can analyze and interpret data, identify patterns, and make informed decisions or predictions based on that analysis.
When machine learning is applied to telematics, it allows for the intelligent analysis of vehicle data to detect patterns and anomalies that may indicate potential issues. By continuously learning and adapting, machine learning algorithms can provide valuable insights and predictions for predictive vehicle maintenance, taking into account various factors such as driving performance and historical data.
How Machine Learning Improves Efficiency in Vehicle Maintenance
Machine learning brings significant improvements to efficiency in vehicle maintenance through its ability to analyze large volumes of data and make accurate predictions. By continuously learning from the data it receives, machine learning algorithms can identify patterns and anomalies that may indicate potential issues or failures.
Traditionally, vehicle maintenance has often been performed based on fixed schedules or reactive responses to unexpected breakdowns. This approach is costly and inefficient, as it does not take into account the actual condition of the vehicle. Machine learning in telematics changes this by enabling predictive maintenance. By analyzing real-time and historical data, machine learning algorithms can detect early warning signs of potential problems, allowing for proactive intervention.
Predictive maintenance not only reduces the risk of unexpected breakdowns but also improves the efficiency of maintenance operations. Instead of servicing vehicles based on arbitrary intervals, resources can be allocated based on actual need. This eliminates unnecessary maintenance tasks and reduces the overall cost of maintenance operations. Additionally, by addressing potential issues before they escalate, the downtime caused by breakdowns can be minimized, leading to improved operational efficiency.
Furthermore, machine learning algorithms can optimize the scheduling of maintenance tasks based on various factors such as engine health data, vehicle usage patterns, and historical maintenance data. By considering these factors, maintenance teams can prioritize vehicles that require attention, ensuring that resources are allocated efficiently. This not only improves the overall efficiency of maintenance operations but also extends the lifespan of vehicles by addressing issues before they lead to major failures.
In summary, machine learning improves efficiency in vehicle maintenance by enabling proactive and predictive maintenance, optimizing resource allocation, reducing unnecessary maintenance tasks, and minimizing downtime caused by breakdowns.
The Role of Data in Machine Learning for Telematics
Data plays a vital role in machine learning for telematics. The effectiveness of machine learning algorithms depends on the quality and quantity of data available for analysis. Telematics systems generate a vast amount of data from various sources, including vehicle sensors, GPS, and driver behavior monitoring.
This data is collected and transmitted to a central system, where it is processed and analyzed by machine learning algorithms. The algorithms identify patterns and anomalies in the data, which are then used to make predictions or decisions. The more data available for analysis, the better the accuracy and reliability of the predictions.
Data in machine learning for telematics can be categorized into two main types: real-time data and historical data. Real-time data refers to the data collected from vehicles in real-time, providing up-to-date information on vehicle performance, driving behavior, and environmental conditions. Historical data, on the other hand, refers to the data collected over a period of time, providing insights into long-term trends and patterns.
Both real-time and historical data are crucial for machine learning algorithms to make accurate predictions. Real-time data allows for immediate detection of anomalies and potential issues, enabling proactive intervention. Historical data provides insights into long-term trends and patterns, allowing for the identification of recurring issues and the development of more accurate predictions.
To ensure the quality of the data, it is important to have proper data collection and storage mechanisms in place. This includes ensuring data accuracy, completeness, and integrity. Additionally, data security and privacy measures should be implemented to protect sensitive information.
In conclusion, data plays a crucial role in machine learning for telematics. The availability of high-quality data is essential for the accurate analysis and prediction of potential vehicle issues, enabling proactive and predictive maintenance.
Implementing Machine Learning in Telematics: Challenges & Considerations
Implementing machine learning in telematics for predictive vehicle maintenance comes with its own set of challenges and considerations. While the benefits are significant, there are several factors that need to be taken into account to ensure successful implementation.
One of the key challenges is data quality and availability. Machine learning algorithms rely on large volumes of high-quality data for accurate predictions. However, ensuring data quality and availability can be a complex task. Data collected from different vehicles may vary in terms of quality, completeness, and accuracy. Additionally, data storage and retrieval processes need to be efficient to handle the large volumes of data generated by telematics systems. Telematics providers have addressed this challenge by offering cloud services with scalable data retrieval and storage from leading cloud solutions such as Amazon Web Services (AWS), Microsoft Azure, Google Cloud.
Another challenge is the need for skilled personnel who can develop, implement, and maintain machine learning algorithms. Machine learning is a specialized field that requires expertise in data analysis, algorithm development, and system integration. Organizations either need to invest in training and hiring skilled professionals to successfully implement machine learning in telematics or partner with telematics providers that have this expertise.
Data security and privacy are also important considerations when implementing machine learning in telematics. Telematics systems collect and transmit sensitive data, including vehicle performance, driver behavior, and location information. Organizations or their telematics partners must have robust security and data privacy measures in place to protect this data from unauthorized access or breaches.
Finally, there is a need for ongoing monitoring and evaluation of machine learning algorithms. As the algorithms learn and adapt over time, their performance needs to be continuously monitored to ensure accuracy and reliability. Feedback loops should be established to incorporate new data and improve the algorithms based on real-world experiences.
Despite these challenges, the benefits of implementing machine learning in telematics for predictive vehicle maintenance outweigh the drawbacks. With careful planning, coordination, and investment, organizations can harness the power of machine learning to drive efficiency and improve maintenance operations.
Future Trends and Advancements in Machine Learning for Telematics
The field of machine learning for telematics is rapidly evolving, with new advancements and trends shaping the future of predictive vehicle maintenance. Here are some key areas to watch out for:
- Advanced anomaly detection: Machine learning algorithms are becoming more sophisticated in detecting anomalies and potential issues in vehicle data. As algorithms learn from more data, they can identify subtle patterns and abnormalities that may indicate impending failures, allowing for even more accurate predictions and proactive maintenance.
- Predictive parts management: Machine learning algorithms can be used not only to predict potential failures but also to optimize parts management. By analyzing historical data, algorithms can predict the lifespan of various vehicle components and recommend proactive replacement or maintenance. This can help organizations optimize parts inventory, reduce downtime, and improve cost-efficiency.
- Enhanced driver behavior monitoring: Machine learning algorithms can analyze driver behavior data to identify patterns and trends that may impact vehicle performance and maintenance needs. By providing real-time feedback and recommendations, algorithms can help drivers adopt safer and more efficient driving habits, reducing vehicle wear and tear and improving overall fleet performance.
These future trends and advancements highlight the continued growth and potential of machine learning in telematics for predictive vehicle maintenance. As technology continues to evolve, organizations can expect even more efficient and cost-effective maintenance operations, leading to improved operational efficiency and enhanced vehicle availability and reliability.
Conclusion
Telematics systems are harnessing the power of machine learning to transition vehicle maintenance from reactive to predictive. By analyzing vast amounts of real-time and historical data, machine learning algorithms can detect patterns and anomalies that may indicate potential vehicle issues. This enables proactive and predictive maintenance, reducing the risk of unexpected breakdowns and optimizing resource allocation.
The benefits of machine learning in telematics for predictive vehicle maintenance are significant. It improves efficiency by enabling proactive intervention, optimizing vehicle servicing schedules, and reducing unnecessary maintenance tasks. It enhances vehicle reliability and safety by analyzing various factors that contribute to wear and tear. It also helps organizations optimize fleet operations and improve overall operational efficiency.
While there are challenges and considerations in implementing machine learning in telematics, organizations can overcome them with proper planning, coordination, and investment and by partnering with companies that are already using machine learning in their telematics solutions.
As the field continues to evolve, future trends and advancements in machine learning for telematics hold great promise. Advanced anomaly detection, predictive parts management, and enhanced driver behavior monitoring are just a few of the areas to monitor going forward.
About Positioning Universal
Established in 2013, Positioning Universal is the leading global provider of off-the-shelf and customizable IoT devices, along with GPS-based monitoring solutions for vehicles and assets. Our Systems Integration (SI) services deliver turn-key solutions for smooth IoT implementations, leveraging our team’s extensive industry knowledge. With a deep understanding of IoT technologies, we guide companies in designing and deploying IoT solutions that meet their unique needs. Our comprehensive offerings, paired with best-in-class customer support, empower businesses with essential business intelligence to sustain a competitive edge in rapidly evolving markets.
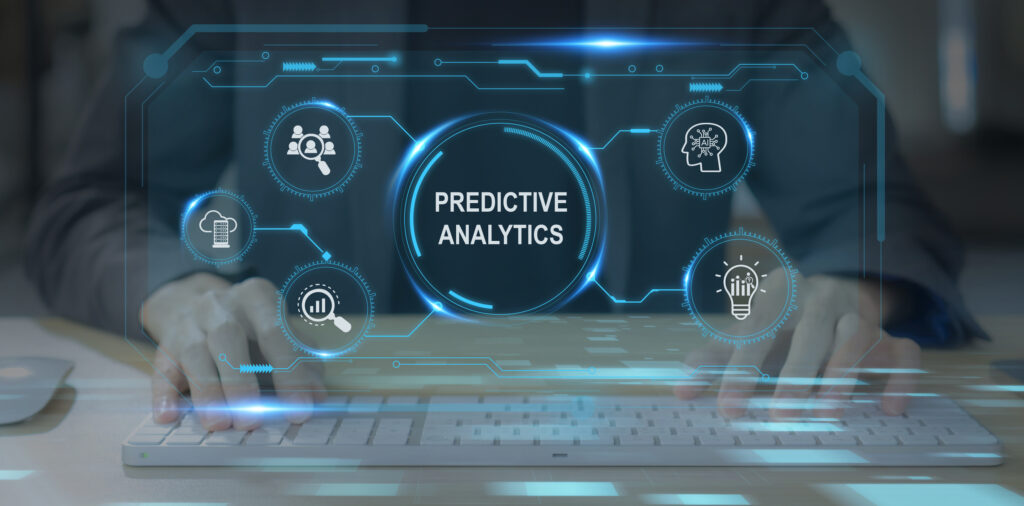